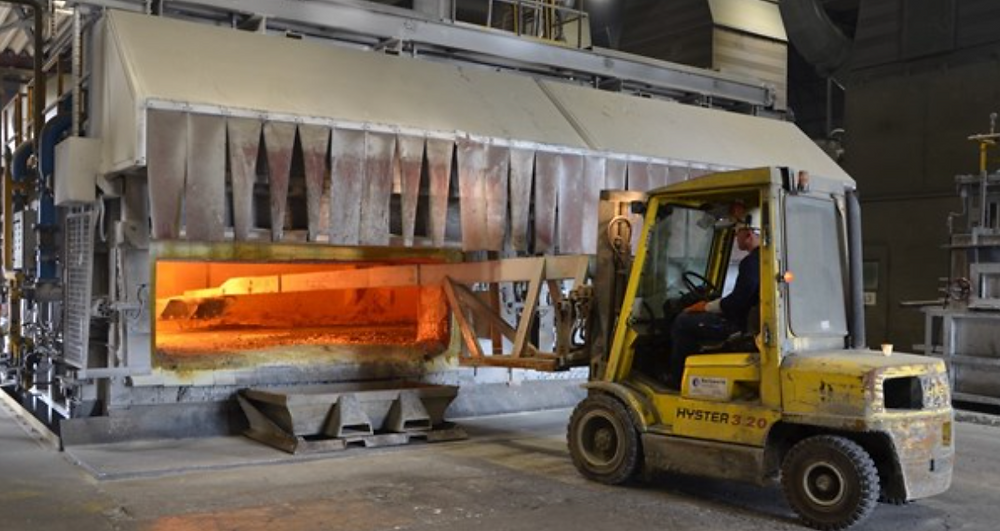
AI in Production Environments: Realism, Statistics and Value Creation
Apr 14
2 min read

Artificial Intelligence (AI) dominates the headlines these days. But what does it actually mean for production and process industries? Many people associate AI with self-learning robots or language models like ChatGPT. In reality, the greatest value for industrial companies lies in something far more tangible: statistical methods – tried, tested, and readily available today.
At Mikon, we help industrial companies gain control of production data, uncover meaningful patterns, reduce losses, and optimise operations. Together with partners such as Visplore, we apply tools and methods often grouped under the AI umbrella – but in practice, they are rooted in solid, practical statistics and data analysis.
What Kind of “AI” Is Actually Relevant?
When people think of AI, they often imagine language models trained to write, translate, or respond to questions. These models are based on vast text datasets and are primarily used in communication – not in process plants or on production lines.
In industry, statistical AI methods are the ones that truly matter. These techniques have existed for decades, but have gained new relevance thanks to better tools, more data, and stronger computing power. Here are a few examples:
Machine Learning: Algorithms that identify patterns in data, for instance between temperature and product quality. Used for prediction and classification.
Statistical Process Control (SPC): Real-time monitoring of data variability to detect issues before they cause major problems.
Correlation Analysis: Identifying which process parameters truly affect product outcomes.
Predictive Modelling: Using historical data to forecast what might happen next.
Anomaly Detection: Automatically flagging unexpected changes or patterns in the data.
Real-World Examples
At Mikon and among our customers, we increasingly see practical applications of statistical AI:
Correlation analysis to understand why quality fluctuates between shifts.
Machine learning to predict when heat exchangers need cleaning.
Predictive alerts for process deviations using SPC and trend analysis.
Each of these use cases leads to measurable improvements – less waste, higher product quality, and lower energy consumption.
Visplore: A Practical AI Tool for Analysis
Our partner Visplore provides a wide range of techniques that qualify as AI – but in reality, they are advanced statistics presented in a highly accessible way. Learn more at visplore.com/features/analytics, where techniques such as clustering, trend analysis, and correlation matrices are made available with no need for programming.
For many industrial organisations, this is the ideal starting point for AI: intuitive, visual tools that help you make use of the data you already have.
What Should You Do Next?
Start with your process data. Do you have easy access to logs, real-time signals, and reports?
Explore patterns and relationships. What drives quality, efficiency, and downtime?
Try simple predictive models. There are low-barrier tools available to get started.
Think evolution, not revolution. You don’t need a grand AI strategy – you need useful insights.
In Summary
AI in industry is not about futuristic robots – it’s about the practical application of statistical methods. It’s about doing more with the data you already have, using tools and techniques that are understandable, effective, and ready to deliver real value.
At Mikon, we’re happy to support you – whether you’re looking to run a pilot project, explore data analysis tools, or just start a conversation about your opportunities.